MLOps, or Machine Learning Operations, is essential for unlocking AI’s full potential by streamlining the deployment, management, and scaling of machine learning models. It integrates data science with IT operations, enabling continuous integration, delivery, and automation of ML pipelines. By improving collaboration, reducing bottlenecks, and ensuring models perform consistently in production, MLOps helps organizations harness the true power of AI to drive innovation, efficiency, and better decision-making across various industries.
What is Machine Learning?
Machine learning is a field of artificial intelligence (AI) that helps computers to gain insights from data and make decisions or predictions without being absolutely programmed.
● It involves the use of algorithms that identify patterns in data and improve performance over time as more data becomes available. Machine learning is used in various applications.
● Machine learning models are trained on very big datasets, enabling them to learn from historical data and make predictions or classify new data accurately.
● To efficiently manage and scale machine learning models in production, organizations use MLOps, a set of practices that bridges the gap between data science and operations. An MLOps course teaches professionals how to automate and optimize the deployment of ML models, ensuring smoother workflows, faster iterations, and better model performance in real-world applications.
How Does Machine Learning Operations Work?
Machine Learning Operations (MLOps) is the practice of integrating machine learning models into production environments efficiently and reliably. It combines data science, software engineering, and IT operations to streamline the lifecycle of ML models, from development to deployment and maintenance. Here’s how it works:
1. Model Development: Data scientists build and train machine learning models using data. This stage involves selecting algorithms, preprocessing data, and training models.
2. Continuous Integration (CI): MLOps ensures that the model development process integrates with version control systems. Any changes to data, code, or model configurations are tracked, enabling consistent updates.
3. Continuous Delivery (CD): Once a model is trained, it is automatically packaged and deployed into production environments. MLOps pipelines handle the model deployment, making it easy to push updates with minimal manual intervention.
4. Monitoring and Maintenance: After deployment, MLOps tools monitor model performance in real-time, tracking metrics like accuracy and response time. This ensures that models perform as expected and flags issues like data drift.
5. Automation: MLOps automates the retraining of models as new data becomes available, ensuring they stay relevant and accurate over time.
6. Collaboration: MLOps fosters collaboration between data scientists, developers, and IT teams, ensuring seamless integration between machine learning and production systems.
By automating these processes, MLOps increases efficiency, reduces errors, and ensures consistent machine learning model performance in production environments.
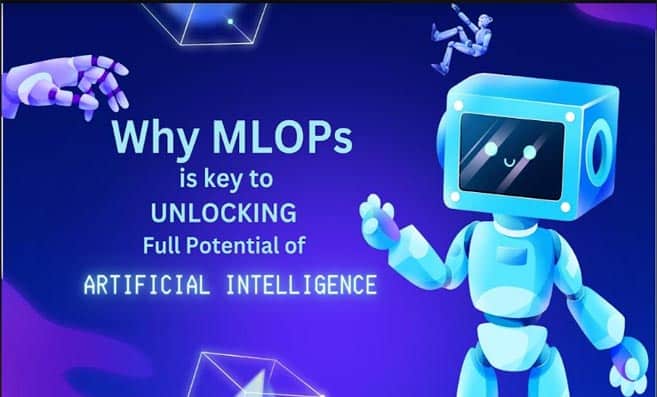
Why MLOps Matters?
MLOps matters because it bridges the gap between data science and IT operations, ensuring the seamless utilization and management of machine learning models in production environments. It enables organizations to automate, monitor, and scale models efficiently, reducing manual intervention and operational bottlenecks. By integrating continuous integration, delivery, and automated workflows, MLOps ensures models remain accurate and up-to-date over time. It also enhances collaboration between data science and engineering teams, improving model reliability and performance. Ultimately, MLOps is critical for businesses to maximize the value of AI and machine learning, driving faster, more informed decision-making and innovation.
How Mlops Help in Unlocking the Full Potential of AI
MLOps helps unlock AI’s full potential by automating and streamlining the deployment, management, and monitoring of machine learning models. By integrating machine learning workflows with IT operations, MLOps ensures that models move seamlessly from development to production, allowing for continuous delivery and faster updates.
MLOps also enables efficient scaling of AI solutions, allowing organizations to handle large datasets and complex models across various environments. Automated monitoring ensures that models are performing optimally and flags issues like data drift. Additionally, MLOps fosters collaboration between data scientists, developers, and operations teams, ensuring smooth transitions from model creation to deployment.
By investing in MLOps training, professionals can acquire the essential skills to manage and optimize AI workflows, making AI-driven solutions more robust, reliable, and scalable, thereby fully realizing the potential of machine learning and AI technologies.
Summed up
In conclusion, MLOps is essential for unlocking the full potential of AI by ensuring the efficient positioning,scaling, and management of machine learning models in production. By automating workflows, enabling continuous integration and delivery, and monitoring model performance, MLOps reduces operational friction and enhances collaboration between data science and IT teams. It helps models stay relevant, scalable, and reliable over time, allowing businesses to maximize their AI investments. Ultimately, MLOps enables organizations to drive innovation, improve decision-making, and harness the full power of AI for real-world applications, making it a critical component of modern AI strategies.